Case Study: Optimizing EHR Data Completeness with On-Premise AI
This case study evaluates the impact of leveraging free-text data from electronic medical records (EMRs) using large language models (LLMs) to enhance the completeness and validity of medication-use information. Using aspirin intake during pregnancy as a case study, we demonstrate how excluding unstructured data sources leads to significant gaps in data completeness, underscoring the critical role of AI-powered data extraction in real-world evidence generation.
This study was honored with the “Best Podium Research Presentation” award at ISPOR Europe 2024.
This case study provides insight into:
- Gaps in EMR data completeness when unstructured sources are excluded
- AI and NLP’s role in enhancing medication-use records
- Aspirin intake patterns in pregnancy
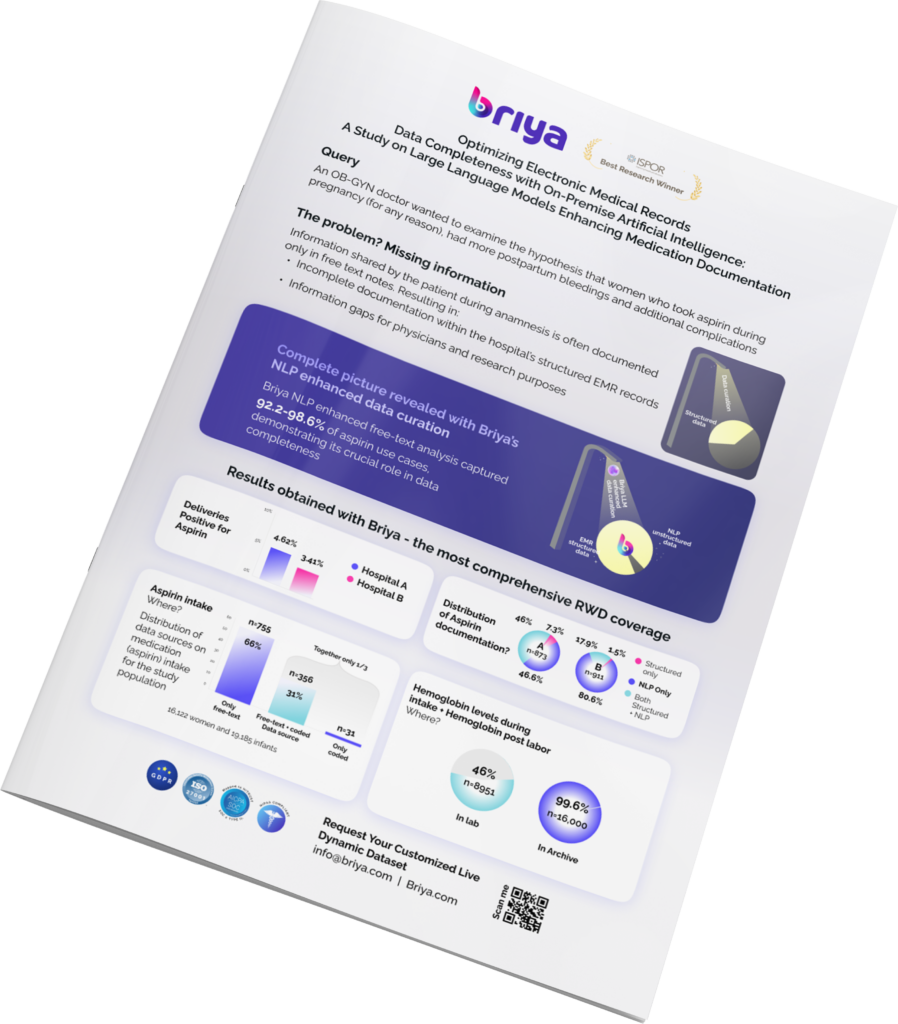